A Primer on AI in Medicine
Medicine is fast approaching a revolution in how it processes information and handles knowledge. This revolution is driven by advances in artificial intelligence (AI). These tools have the potential to reshape the way we work as physicians. As such, it is imperative that we, as physicians, equip ourselves with the knowledge to navigate and lead in this new terrain. My journey through the MD-PhD program at the University of Michigan has afforded me a unique vantage point at the intersection of clinical practice and AI research. My experience makes me excited about the potential and weary about the potential risks.
Ultimately, successfully integrating technology in medicine requires a deep understanding of human and technical factors. My previous work on bringing human factors into primary care policy and practice discusses how a deeper study of human factors is needed to make technology more impactful in healthcare. While we still need this deeper work, more minor changes to how we train physicians can also have a significant impact. Often, critical problems at the interface between physicians and technology aren’t addressed because physician users are not empowered to ask the right questions about their technology. This “chalk talk” was initially designed to encourage fourth-year medical students to feel comfortable asking several fundamental questions about the AI tools they may be confronted with in practice.
In addition to recording a slideshow version of the chalk talk, I have also taken the opportunity to write more about the topic and have included materials for medical educators looking to deliver or adapt this chalk talk.
The Surge of AI in Healthcare
The healthcare industry has witnessed an unprecedented surge in AI model development in recent years. The FDA certified its 692nd AI model in 2023, a significant leap from the 73 models certified less than five years prior. This rapid growth underscores the increasing role of AI in healthcare, from diagnostics to treatment and operations. However, it’s crucial to recognize that many AI models bypass FDA certification, as they primarily serve as decision-support tools for physicians rather than direct diagnostic tools. For example, many of the models produced by vendors, like the Epic sepsis model, and internal models produced by health systems and their researchers do not need FDA certification. Together, these models constitute the vast majority of the healthcare AI iceberg.
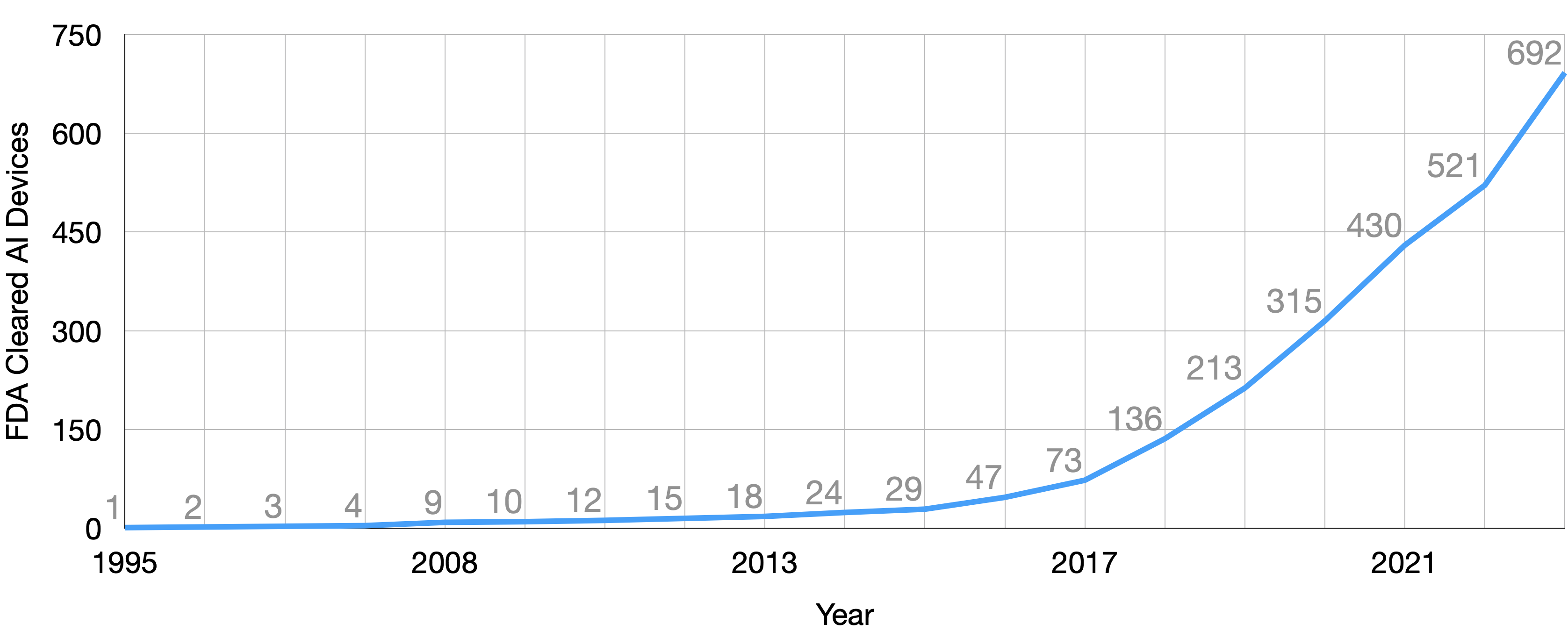
Demystifying AI: Key Questions for Physicians
As AI models become more integrated into our clinical workflows, understanding the fundamentals of these tools is crucial. Here are three key questions every physician should feel comfortable asking:
- How was it made?
- Is it any good?
- How is it being used?
Now that we’ve laid out the essential questions to consider, let’s unpack each question and introduce relevant technical definitions to facilitate communication between physicians and engineers.
How Was It Made? Medical AI Model Development
The creation of an AI model is called development by engineers. This term encompasses creating an AI model, starting with the initial definition of the clinical problem and ending with the finished model ready for use. The fundamental goal of model development is to create a model, a function that can estimate unknown outcomes using known information.
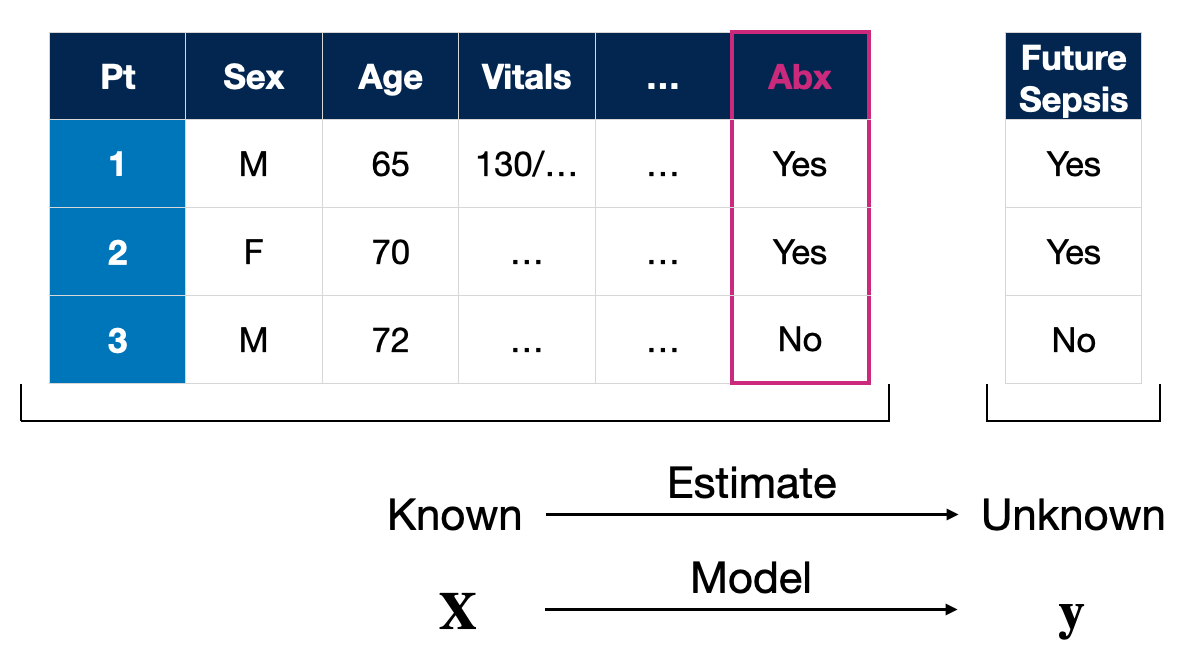
The Foundation of Data
Data is the foundation of most AI models. Although AI tools can be built without data, most advanced tools used in healthcare are derived from large amounts of data. The type and quality of this data are paramount. In healthcare, much of this data is gathered retrospectively, meaning we look back at what has already happened to understand and predict what may happen in the future. This historical data is used to develop and validate our models. In the development process, we hope to learn a model that picks up on essential signals, patterns, and relationships hiding in this data.
Known vs. Unknown: The \(\bf{X}\) and \(\bf{y}\) of AI
In supervised machine learning, the most common AI approach in medicine, data is divided into two main categories: known data and unknown data. Known data, \(\bf{X}\), encompasses the variables we know or can measure/collect directly—age, vital signs, medical comorbidities, and treatments given are some examples. These known variables are what the model uses to make predictions or estimates about unknown variables, \(\bf{y}\), which could be future clinical events such as the onset of sepsis.
NB: You may see \(\bf{x}\) and \(y\) used to represent the information for individual patients in other places, like papers or textbooks. The notation I’m using represents a population or group of patients. Just for completion sake, the model is a function that maps these to one another, \(f(\bf{X}) \to \bf{y}\). Don’t sweat the notation details; save that for my forthcoming textbook. :p
The Crucial Role of Clinical Insight
Clinical perspective is essential during model development to ensure relevance and utility. Clinicians provide the context that is critical for interpreting data correctly. Without their input, a model might include variables that, while statistically significant, could lead to incorrect or late predictions.
For example, consider a sepsis prediction model that uses fluid and antibiotic administration data. These factors are indicators of resuscitation efforts already in progress. If the model relies too heavily on these markers, its alerts would only confirm what clinicians have already recognized rather than providing an early warning.
This reinforces a critical point in model development: if a model inadvertently includes data directly tied to the outcome it’s predicting, it can lead to a decrease in clinical utility. There’s evidence of AI models in healthcare that, unintentionally, have relied on such information, thus reducing their effectiveness in real-world settings.
As physicians, it’s part of our responsibility to probe these models, ask the right questions, and ensure they provide the maximum clinical benefit.
Is It Any Good? Validation of Healthcare AI
The question of clinical benefit naturally brings us to the second question: are the models any good? There are many dimensions to measure if an AI model is any good. However, the first place to start is from a technical/statistical point of view. Engineers often refer to this assessment as validation.
In the validation phase we typically scrutinize the model’s performance on fresh data, data it hasn’t previously ‘seen.’ Some of the ideas underpinning validation should be familiar to physicians as we apply concepts from evidence-based medicine and biostatistics to measure the performance of AI models.
Validation involves testing the AI model against a data set that was neither used in its training nor influenced its development process. This could include data deliberately set aside for testing (a test dataset) or new data encountered during the model’s actual use in clinical settings (data collected during prospective use).
Why Validate with Unseen Data?
The reason behind using unseen data is simple: to avoid the trap of overfitting. Overfitting occurs when a model, much like a student who crams for a test, ‘memorizes’ the training data so well that it fails to apply its knowledge to new, unseen situations.
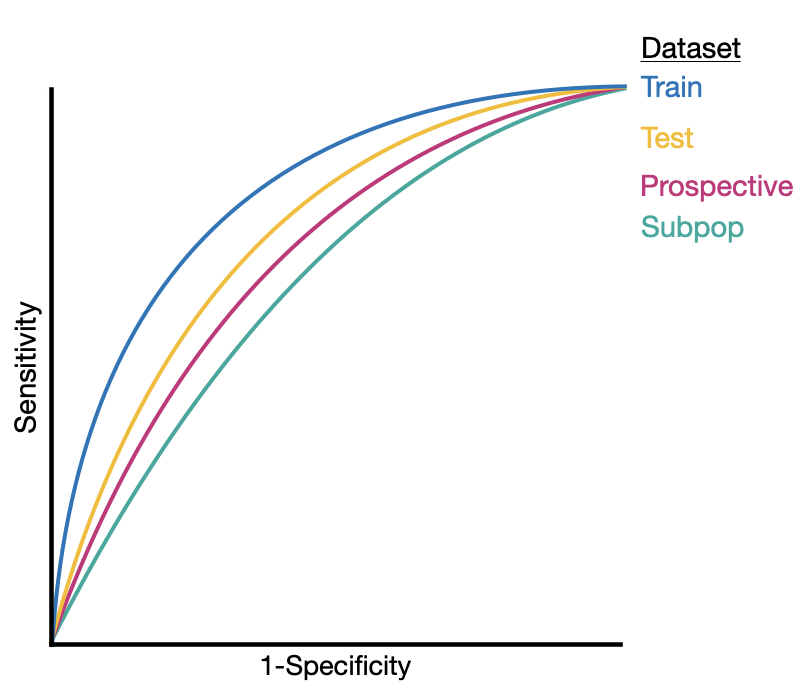
Performance Across Different Populations
Another aspect of validation is assessing model performance across various populations. Models optimized for one group may not perform as well with others, especially vulnerable subpopulations. These groups may not be represented adequately in the training data, leading to poorer outcomes when the model is applied to them.
Even subtle differences in populations can lead to degradation in AI model performance.
The Metrics That Matter
As mentioned above, validation mirrors evidence-based medicine/biostatics. Measures from those fields, like sensitivity, specificity, accuracy, positive predictive value (PPV), negative predictive value (NPV), number needed to treat (NNT), and the area under the receiver operating characteristic curve (AUROC), along with measures of calibration are often used to measure AI model performance formally.
Ongoing Validation: A Continuous Commitment
Validation shouldn’t be viewed as a one-time event. It’s a continuous process that should accompany the AI model throughout its existence, from development to integration into care processes. Most importantly, AI models should continually be assessed throughout their use in patient care. We can adjust and refine the model’s performance by continually reassessing it, ensuring it remains a reliable tool in a clinician’s arsenal. If it no longer meets clinical needs or performs safely, we can swiftly remove or replace it.
The validation of an AI model in healthcare is more than a technical requirement—it’s a commitment to patient safety and delivering high-quality care. By rigorously evaluating AI tools against these standards, we can ensure that these technologies serve their intended purpose: supporting and enhancing the medical decisions that affect our patients’ lives.
How Is It Being Used? Implementation into Clinical Workflows
The final aspect you should consider asking about a medical AI system concerns its application — how is the AI system utilized in clinical settings? Implementation is the phase where the model is transitioned from bench to bedside. It involves connecting the theoretical capabilities of AI models to the practical day-to-day operations in healthcare environments.
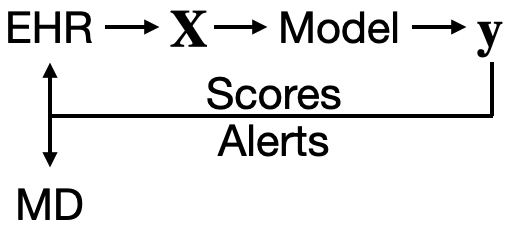
The Integration of AI with Clinical Workflows
AI’s integration into clinical workflows should be designed with the following objective: to provide timely, relevant information to the right healthcare provider. Let’s consider a typical workflow involving AI. It begins with electronic health records (EHRs) supplying the raw data. This data is then processed into input variables, \(\bf{X}\), which feed into the AI model. The model processes these inputs and outputs a prediction, \(\bf{y}\), which could relate to patient risk factors, potential diagnoses, or treatment outcomes.
From Data to Decision: The Role of AI-Generated Scores and Alerts
The scores generated by the model can be channeled back into the EHR system, presenting as risk scores for a list of patients that clinicians can review and sort by. Alternatively, they can be directed straight to the physicians in the form of alerts — perhaps as a page or a prompt within the EHR system. These alerts are intended to be actionable insights that aid medical decision-making.
The Crucial Feedback Loop with Clinicians
Acknowledging that AI developers may not have an intricate understanding of clinical workflows is imperative. This gap can lead to alerts that, despite their good intentions, may add little value or disrupt clinical processes. If alerts are poorly timed or irrelevant, they risk becoming just another beep in a cacophony of alarms, potentially leading to alert fatigue among clinicians.
The Empowerment of Physicians in Implementation
As physicians, it is within our purview — indeed, our responsibility — to demand improvements when AI tools fall short. With a deep understanding of the nuances of patient care, we are in a prime position to guide the refinement of these tools. If an AI-generated alert does not contribute to medical decision-making or interrupts workflows unnecessarily, we should not hesitate to call for enhancements.
The successful implementation of AI in medicine is not a one-way street where developers dictate the use of technology. It is a collaborative process that thrives on feedback from clinicians. By actively engaging with the development and application of AI tools, we ensure they serve as a beneficial adjunct to the art and science of medicine rather than a hindrance.
AI in Medical Education: Fostering AI Literacy
Its not a question of if we need to teach AI in medicine, its is a question of when (NOW!) and how. I believe incorporating discussions on AI into medical education is imperative for preparing future physicians. Educators should strive to create an environment that encourages critical thinking about the role of technology in medicine. This includes understanding how AI models are built and validated and contemplating their ethical implications and potential biases. We can start a more meaningful dialogue between physicians and engineers by equipping all physicians with these three questions.
If you are a medical educator, consider adding this content to your curriculum. You can directly assign the video or give your rendition of the chalk talk live. Here’s a teaching script you can use directly or adapt.
Looking Ahead
As we navigate the AI-driven healthcare transformation, our approach must be guided by enthusiasm for innovation and a rigorous commitment to patient care. By fostering a deep understanding of AI among medical professionals and integrating these discussions into medical education, we can ensure that the future of healthcare is both technologically sound and fundamentally human-centered.
I invite you to engage in this vital conversation, share your thoughts, and explore how we can collectively harness the power of AI to improve patient outcomes. For further discussions or inquiries, feel free to connect by email.
Cheers,
Erkin
Go ÖN Home